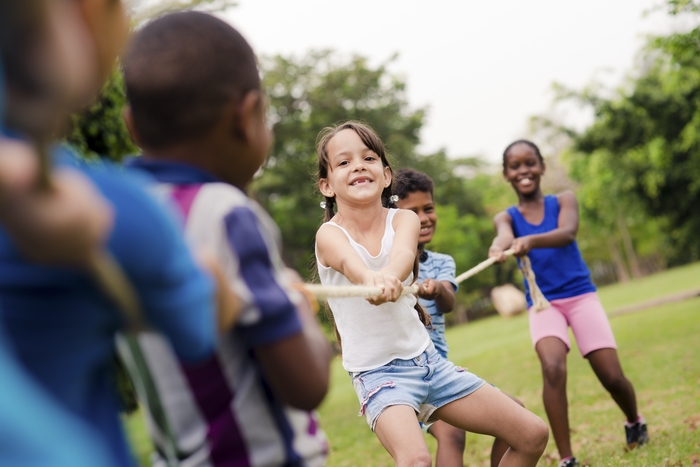
A team led by researchers at Weill Cornell Medicine has used an AI-based approach to uncover underlying patterns among the conditions in which people are born, grow, live, work, and age, termed social determinants of health (SDoH), and then linked each pattern to children’s health outcomes. Compared with traditional approaches, the strategy, in principle, provides a more objective and comprehensive picture of potential social factors that affect child health, which in turn, can enable better targeted interventions.
As reported Oct. 16 in JAMA Pediatrics, the researchers analyzed data on more than 10,500 American children, in communities across 17 U.S. states. Quantifying more than 80 neighborhood-level SDoH factors for each child, the analysis uncovered four broad patterns in the sample, including affluence, high-stigma environment, high socioeconomic deprivation, and high crime and drug sale rates coupled with lower education and densely populated areas. They found statistical associations between these patterns and outcomes relating to child developmental health, including mental, cognitive and physical health.
“A complex set of social factors can influence children’s health, and I think our results underscore the importance of using methods that can handle such complexity,” said study lead author Dr. Yunyu Xiao, an assistant professor of population health sciences at Weill Cornell Medicine.
Dr. Xiao co-led the study with Dr. Chang Su, also an assistant professor of population health sciences. Both are in the Division of Health Informatics in the Department of Population Health Sciences at Weill Cornell Medicine. Dr. Jyotishman Pathak and Dr. Fei Wang, also at Weill Cornell Medicine, are co-authors in this joint work.
The Weill Cornell Medicine investigators work with a multi-institutional, multidisciplinary team of experts to study potential social determinants of health for clues to persistent causes of bad health outcomes. The team includes psychiatry expert Dr. John Mann from Columbia University; Drs. Timothy Brown, Lonnie Snowden, and Julian Chun-Chung Chow, experts in health economics, health policy and social welfare, respectively, at the University of California; Berkeley School of Public Health, and social epidemiologist Dr. Alex Tsai of Harvard Medical School. Identifying health-influencing social factors also can guide social policies aimed at improving child health, such as legislation mandating free school lunches for children from low-income families coupled with holistic health care provisions at school and clinical settings, Dr. Xiao said.
A New Approach to a Complex Issue
Prior studies in this field have tended to focus on narrow sets of socioeconomic variables and health outcomes, and typically have examined outcomes that are averaged over large geographic areas such as counties or states.
In the new study, the researchers took a different approach. Drs. Xiao and Su are experts in the use of machine learning and other advanced AI techniques that allow relatively unbiased, fine-grained analyses of large datasets. In recent years, they have been bringing these “big-data” techniques to bear on important social epidemiology problems—for example, examining factors potentially influencing children’s mental health during the COVID-19 pandemic.
“Our approach is data-driven, allowing us to see what patterns there are in large datasets, without prior hypotheses and other biases getting in the way,” Dr. Su said.
The dataset in the new study was generated by an ongoing, survey-based, National Institutes of Health (NIH)-sponsored project called the Adolescent Brain Cognitive Development (ABCD) Study. It covered a cohort of 10,504 children, aged 9-10 at the start, and their parents at 21 sites across the United States from 2016 to 2021. The sample’s ethnic and racial mix broadly reflected that of the U.S. as a whole.
In the analysis, each child’s record was scored on 84 different SDoH variables relating to educational resources, physical infrastructure, perceived bias and discrimination, household income, neighborhood crime and drugs. The machine learning algorithm identified underlying patterns in the children’s SDoH profiles – and also looked for statistical associations between these patterns and health outcomes.
Child Health Outcomes Vary Depending on Social Determinants
A key finding was that the data clustered into four broad SDoH patterns: affluent; high socioeconomic deprivation; urban high crime and low level of educational attainment and resources; and high-stigma—the latter involving higher self-reported measures of bias and discrimination against women and immigrants and other underrepresented groups. White children were overrepresented in the affluent and high-stigma areas; Black and Hispanic children in the other two.
Each of the four profiles was associated with its own broad pattern of health outcomes, the “high socioeconomic deprivation” pattern being associated with the worst health outcomes on average, including more signs of mental illness, worse cognitive performance, and worse physical health. The other two non-affluent patterns were also associated generally with more adverse outcomes compared with the affluent pattern.
The study had some limitations, including the survey-based, self-reported nature of the ABCD data, which is generally considered less reliable than objectively measured data. Also, epidemiological analyses like these can reveal only associations between social factors and health outcomes—they can’t prove that the former influence the latter. Even so, the researchers said, the results demonstrate the power of a relatively unbiased, machine-learning approach to uncover potentially meaningful links, and should help inform future studies that can discover actual causative mechanisms connecting social factors to child health.
“This multi-dimensional, unbiased approach in principle can lead to more targeted and effective policy interventions that we are investigating in a current NIH-funded project,” Dr. Xiao said.